Feral animal control is a big problem for Australian agriculture. Losses from feral pigs and foxes have been estimated by CSIRO at more than $1b. Since control by baiting causes drastic collateral damage to native species, shooting is the preferred option for control, but the intelligence of many feral animals means that shooters have to be in the right place at the right time to achieve results. Poaching is a smaller scale, but still serious, problem in some areas.
Camera traps (or trail cameras) can be useful for farmers in dealing with both problems. They act like burglar alarms, being triggered by a change in the heat distribution in the sensed area caused by motion of an object in the field of view. Camera traps can record one or more images, or a short video when triggered. Some cameras can even transmit image and video data to a central location. Camera trap data can be used to more profitably deploy shooters and help control poaching.
Historically, all the sensed data has had to be viewed by a human to determine whether the image of video shows an entity of interest, and the frequency of false triggerings caused by moving vegetation or shadows means that thousands of images of videos have to be viewed. However, advances in AI (or more specifically machine vision) mean that fully automated methods can be used to detect entities of interest (animals, people or vehicles) in image and videos. There is even capability for detecting animal species, allowing discrimination between feral animals and native ones, such as marsupials. Whilst AI systems cannot match the peak performance of an alert human and will always miss or misidentify some entities, their ability to run continuously is of great value, as peak human performance cannot be sustained for long enough to process the quantity of data generated by camera traps. AI systems perform particularly well for vehicle detection and can form a key part of an anti-poaching strategy.
Machine vision and AI systems require considerable computer power to run and a computer with a GPU (Graphics Processing Unit) is required to achieve a reasonable processing speed. Computers designed for gaming have such devices and their price is falling due to the spread of AI-based applications.
CamTrap Pro can use results from machine vision programs such as EcoAssist or it can operate using detection algorithms without using machine vision. An example of of non-machine learning detection is shown below
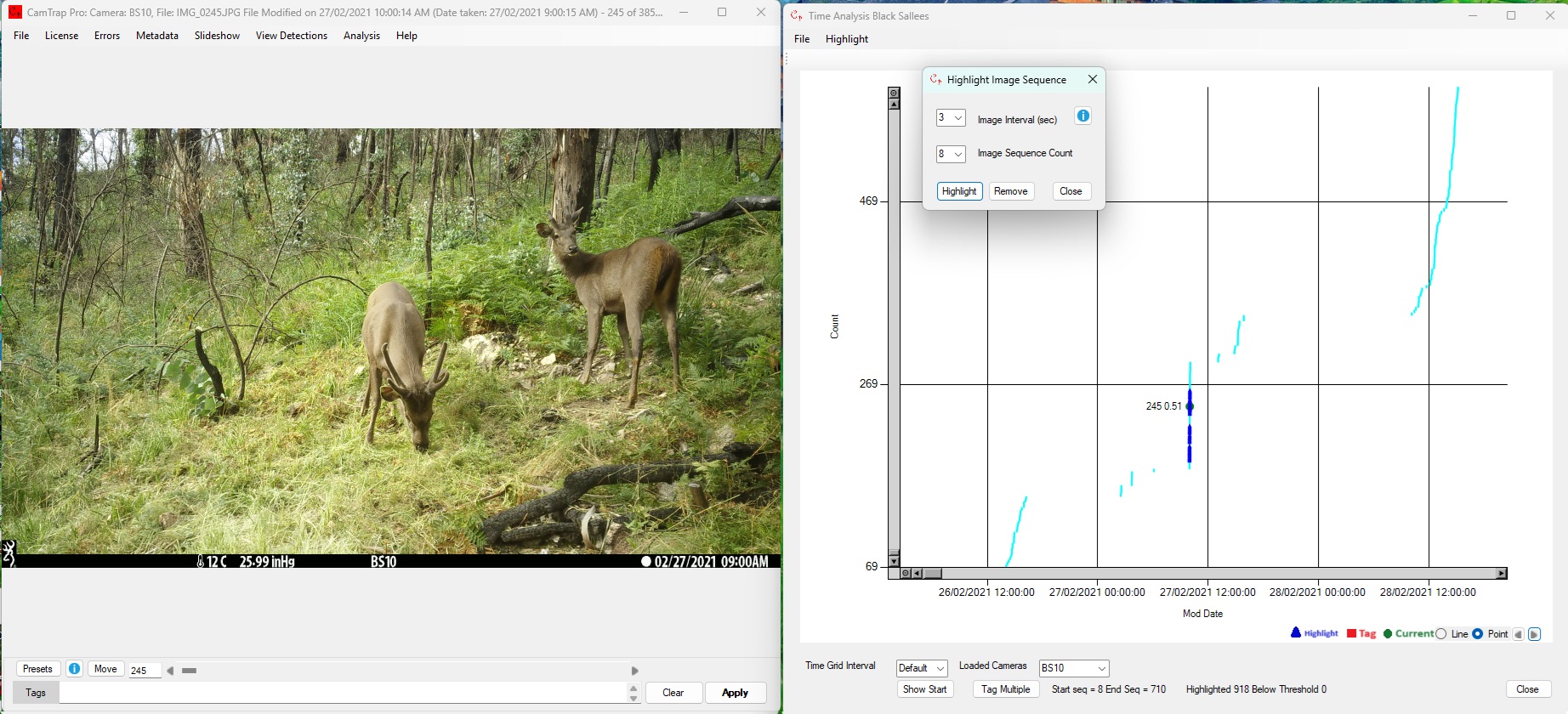
The Count vs Time profile shows the number of images recorded as a function of time. False triggering is typically randomly spaced in time, whereas animal triggerings are typically closely spaced in time (as the animal moves around in the sensed area) and are indicated by a vertical step in the Count vs Time profile. These can easily be detected by a simple, fast algorithm
Machine vision results can also be used for animal detection and species identification. The image below shows results using a species detection model in CamTrap Pro:
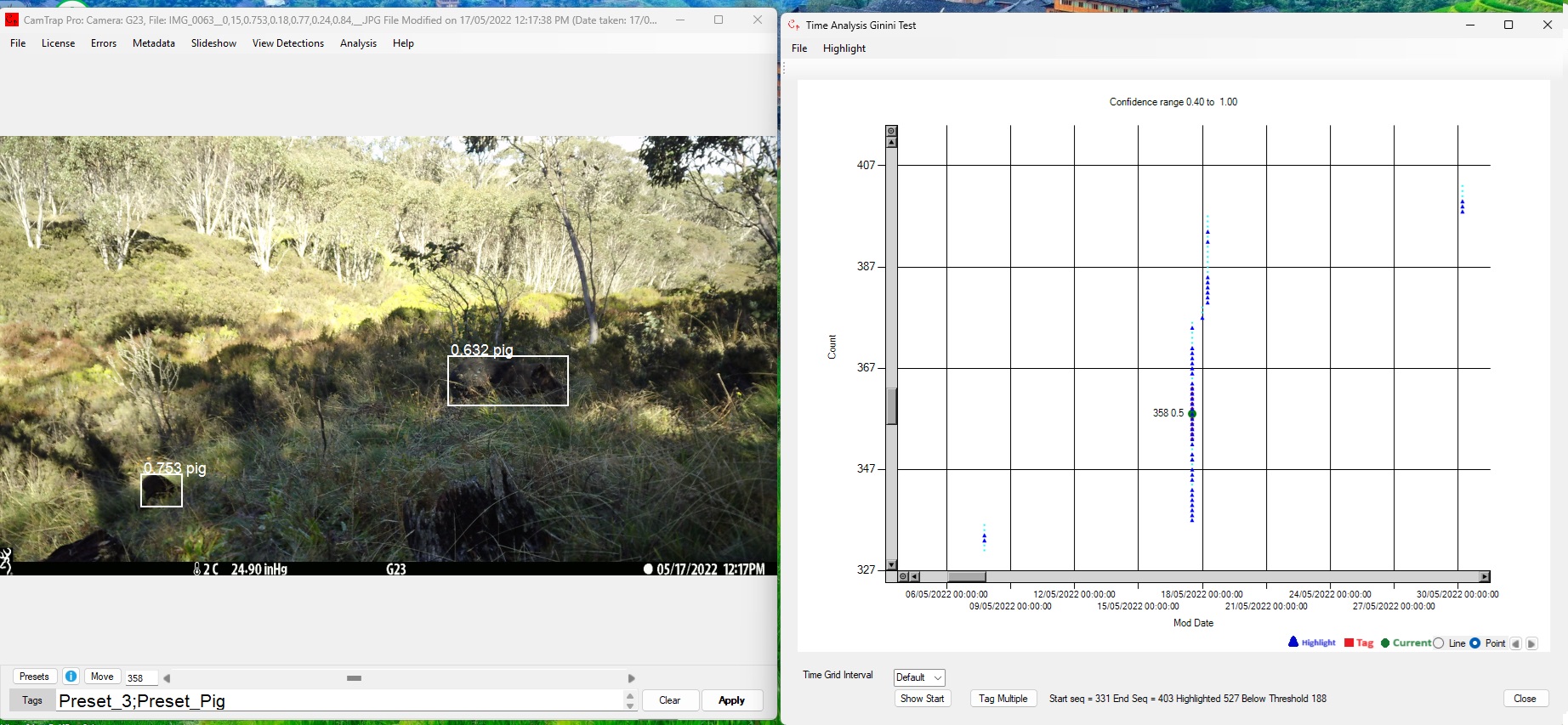
Machine vision highlighting is much more specific than sequence highlighting. It can detect the species of animal present and define a bounding box around it, but uses a great deal more computing power. It will also have less than perfect precision and recall – some animal detections will be missed, others will be misclassified and there will be false detections where an inanimate object is identified as an animal.
For more information on CamTrap Pro click here. Click here to download CamTrap Pro, view video tutorials or read the manual. Downloads come with a free demo license which allows loading of up to 20000 images and lasts for 30 days. Permanent licenses can be purchased from within the application at a cost of US$50 – US$90 depending on the number of files to be processed.